42 learning with less labels
Image Classification and Detection - PLAI The DARPA Learning with Less Labels (LwLL) program aims to make the process of training machine learning models more efficient by reducing the amount of ... Learning with Less Labels Imperfect Data | Hien Van Nguyen Program for Medical Image Learning with Less Labels and Imperfect Data (October 17, Room Madrid 5) 8:00-8:05 8:05-8:45 Opening remarks Keynote Speaker: Kevin Zhou, Chinese Academy of Sciences Keynote Speaker: Pallavi Tiwari, Case Western Reserve University Oral Presentations (6 minutes for each paper)
Learning with less labels in Digital Pathology via Scribble ... - DeepAI Learning with less labels in Digital Pathology via Scribble Supervision from natural images 1 Introduction. Digital Pathology (DP) is a field that involves the analysis of microscopic images. ... Unfortunately,... 3. The function, denotes the segmentation model, indicates the number of classes ...
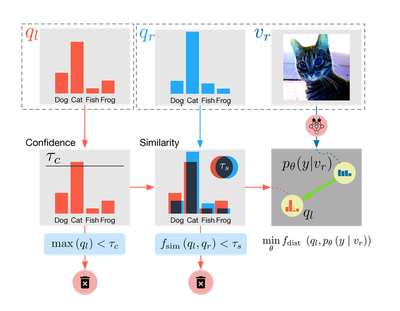
Learning with less labels
Learning With Auxiliary Less-Noisy Labels | Request PDF - ResearchGate However, learning with less-accurate labels can lead to serious performance deterioration because of the high noise rate. Although several learning methods (e.g., noise-tolerant classifiers) have ... Learning with Less Labeling (LwLL) - Zijian Hu The Learning with Less Labeling (LwLL) program aims to make the process of training machine learning models more efficient by reducing the amount of labeled data required to build a model by six or more orders of magnitude, and by reducing the amount of data needed to adapt models to new environments to tens to hundreds of labeled examples. Learning With Less Labels (lwll) - mifasr DARPA Learning with Less Labels (LwLL)HR0Abstract Due: August 21, 2018, 12:00 noon (ET)Proposal Due: October 2, 2018, 12:00 noon (ET)Proposers are highly encouraged to submit an abstract in advance of a proposal to minimize effort and reduce the potential expense of preparing an out of scope proposal.Grants.govFedBizOppsDARPA is soliciting innovative research proposals in the area of machine ...
Learning with less labels. Learning with Fewer Labels in Computer Vision: LwFLCV This special issue focuses on learning with fewer labels for computer vision tasks such as image classification, object detection, semantic segmentation, instance segmentation, and many others and the topics of interest include (but are not limited to) the following areas: • Self-supervised learning methods • New methods for few-/zero-shot learning Less is More: Labeled data just isn't as important anymore Here's one possible procedure (called SSL with "domain-relevance data filtering"): 1. Train a model ( M) on labeled data ( X) and the true labels ( Y). 2. Calculate the error. 3. Apply M on unlabeled data ( X') to "predict" the labels ( Y'). 4. Take any high-confidence guesses from (2) and move them from X' to X. 5. Repeat. Learning with Limited Labeled Data, ICLR 2019 Increasingly popular approaches for addressing this labeled data scarcity include using weak supervision---higher-level approaches to labeling training data ... Learning With Less Labels - YouTube About Press Copyright Contact us Creators Advertise Developers Terms Privacy Policy & Safety How YouTube works Test new features Press Copyright Contact us Creators ...
Learning with Less Labels and Imperfect Data | MICCAI 2020 - hvnguyen Methods such as one-shot learning or transfer learning that leverage large imperfect datasets and a modest number of labels to achieve good performances. Methods for removing rectifying noisy data or labels. Techniques for estimating uncertainty due to the lack of data or noisy input such as Bayesian deep networks. Learning with Less Labeling (LwLL) - Darpa The Learning with Less Labeling (LwLL) program aims to make the process of training machine learning models more efficient by reducing the amount of labeled ... Charles River to take part in DARPA Learning with Less Labels program ... Charles River Analytics Inc. of Cambridge, MA announced on October 29 that it has received funding from the Defense Advanced Research Projects Agency (DARPA) as part of the Learning with Less Labels program. This program is focused on making machine-learning models more efficient and reducing the amount of labeled data required to build models. Learning with Limited Labels | Open Data Science Conference - ODSC Large-scale labeled training datasets have enabled deep neural networks to excel across a wide range of benchmark machine learning tasks. However, in many problems, it is prohibitively expensive and time-consuming to obtain large quantities of labeled data. This talk introduces my recent research on learning with less labels.
Learning With Auxiliary Less-Noisy Labels | IEEE Journals & Magazine ... Learning With Auxiliary Less-Noisy Labels Abstract: Obtaining a sufficient number of accurate labels to form a training set for learning a classifier can be difficult due to the limited access to reliable label resources. Instead, in real-world applications, less-accurate labels, such as labels from nonexpert labelers, are often used. Less Labels, More Learning | AI News & Insights 2 min read. In small data settings where labels are scarce, semi-supervised learning can train models by using a small number of labeled examples and a larger set of unlabeled examples. A new method outperforms earlier techniques. What's new: Kihyuk Sohn, David Berthelot, and colleagues at Google Research introduced FixMatch, which marries two semi-supervised techniques. Darpa Learning With Less Label Explained - Topio Networks The DARPA Learning with Less Labels (LwLL) program aims to make the process of training machine learning models more efficient by reducing the amount of labeled data needed to build the model or adapt it to new environments. In the context of this program, we are contributing Probabilistic Model Components to support LwLL. Learning with less labels in Digital Pathology via Scribble Supervision ... Download Citation | Learning with less labels in Digital Pathology via Scribble Supervision from natural images | A critical challenge of training deep learning models in the Digital Pathology (DP ...
Learning with Less Labels in Digital Pathology via Scribble Supervision ... Learning with Less Labels in Digital Pathology via Scribble Supervision from Natural Images 7 Jan 2022 · Eu Wern Teh , Graham W. Taylor · Edit social preview A critical challenge of training deep learning models in the Digital Pathology (DP) domain is the high annotation cost by medical experts.
Learning With Less Labels (lwll) - beastlasopa Learning with Less Labels (LwLL). The city is also part of a smaller called, as well as 's region.Incorporated in 1826 to serve as a, Lowell was named after, a local figure in the. The city became known as the cradle of the, due to a large and factories. Many of the Lowell's historic manufacturing sites were later preserved by the to create.
Less Labels, More Learning | AI News & Insights How it works:FixMatch learns from labeled and unlabeled data simultaneously. It learns from a small set of labeled images in typical supervised fashion. It learns from unlabeled images as follows: FixMatch modifies unlabeled examples with a simple horizontal or vertical translation, horizontal flip, or other basic translation.
Machine learning with less than one example - TechTalks A new technique dubbed "less-than-one-shot learning" (or LO-shot learning), recently developed by AI scientists at the University of Waterloo, takes one-shot learning to the next level. The idea behind LO-shot learning is that to train a machine learning model to detect M classes, you need less than one sample per class.
Machine learning with limited labels: How to get the most out ... A) Start with an entirely unlabeled dataset: The first step is then to let the domain expert define a set of labeling functions, which are functions that divide up the problem space in our simple example. B) Combine resulting weak labels: This is done by learning the (generative) label model. This model gives us probabilistic labels as output.
subeeshvasu/Awesome-Learning-with-Label-Noise - GitHub 2017 - Learning with Auxiliary Less-Noisy Labels. 2018-AAAI - Deep learning from crowds. 2018-ICLR - mixup: Beyond Empirical Risk Minimization. 2018-ICLR - Learning From Noisy Singly-labeled Data. 2018-ICLR_W - How Do Neural Networks Overcome Label Noise?. 2018-CVPR - CleanNet: Transfer Learning for Scalable Image Classifier Training with Label ...
Learning in Spite of Labels - amazon.com Paperback. $9.59 31 Used from $2.49 1 New from $22.10. All children can learn. It is time to stop teaching subjects and start teaching children! Learning In Spite Of Labels helps you to teach your child so that they can learn. We are all "labeled" in some area. Some of us can't sing, some aren't athletic, some can't express themselves well ...
learning styles: the limiting power of labels There's an ongoing and lively debate in the world of education about the prevalence and relevance of 'learning styles'. Various models try to place a label on how people prefer to learn, and the number of labels within these models also varies widely. In a coordinated effort, the Debunker Club is targeting the 'Learning Styles Myth' throughout the month…
Paper tables with annotated results for Learning with Less Labels in ... Learning with Less Labels in Digital Pathology via Scribble Supervision from Natural Images . A critical challenge of training deep learning models in the Digital Pathology (DP) domain is the high annotation cost by medical experts. One way to tackle this issue is via transfer learning from the natural image domain (NI), where the annotation ...
Learning With Auxiliary Less-Noisy Labels - PubMed The proposed method yields three learning algorithms, which correspond to three prior knowledge states regarding the less-noisy labels. The experiments show that the proposed method is tolerant to label noise, and outperforms classifiers that do not explicitly consider the auxiliary less-noisy labels.
Learn about retention policies & labels to retain or delete - Microsoft ... Retention label policies specify the locations to publish the retention labels. The same location can be included in multiple retention label policies. You can also create one or more auto-apply retention label policies, each with a single retention label. With this policy, a retention label is automatically applied when conditions that you ...
Learning with Less Labels in Digital Pathology via Scribble ... We demonstrate that scribble labels from NI domain can boost the performance of DP models on two cancer classification datasets (Patch Camelyon Breast Cancer and Colorectal Cancer dataset). Furthermore, we show that models trained with scribble labels yield the same performance boost as full pixel-wise segmentation labels despite being significantly easier and faster to collect.
DARPA Learning with Less Labels LwLL - Machine Learning and Artificial ... DARPA Learning with Less Labels LwLL - Machine Learning and Artificial Intelligence Sponsor Deadline: Oct 2, 2018 Letter of Intent Deadline: Aug 21, 2018 Sponsor: DOD Defense Advanced Research Projects Agency UI Contact: lynn-hudachek@uiowa.edu Updated Date: Aug 15, 2018 Email this DARPA Learning with Less Labels (LwLL) HR001118S0044
Learning With Less Labels (lwll) - mifasr DARPA Learning with Less Labels (LwLL)HR0Abstract Due: August 21, 2018, 12:00 noon (ET)Proposal Due: October 2, 2018, 12:00 noon (ET)Proposers are highly encouraged to submit an abstract in advance of a proposal to minimize effort and reduce the potential expense of preparing an out of scope proposal.Grants.govFedBizOppsDARPA is soliciting innovative research proposals in the area of machine ...
Learning with Less Labeling (LwLL) - Zijian Hu The Learning with Less Labeling (LwLL) program aims to make the process of training machine learning models more efficient by reducing the amount of labeled data required to build a model by six or more orders of magnitude, and by reducing the amount of data needed to adapt models to new environments to tens to hundreds of labeled examples.
Learning With Auxiliary Less-Noisy Labels | Request PDF - ResearchGate However, learning with less-accurate labels can lead to serious performance deterioration because of the high noise rate. Although several learning methods (e.g., noise-tolerant classifiers) have ...
Post a Comment for "42 learning with less labels"